By doing so, Wienerberger has become capable to perform smart energy management at that plant, which resulted in a significant reduction of their operational costs and carbon footprint, while also allowing to scale this solution to other plants.
About Wienerberger
Wienerberger is a leading manufacturer of building materials with its headquarters based in Austria. Since producing roof tiles and bricks is part of their core business, they have their own manufacturing facilities that are responsible for this, including 11 brick factories in Belgium. Next to that, three of the most important pillars of Wienerberger’s strategy for 2023 are ESG, innovation, and operational excellence. In order to achieve the sustainable growth they aim for, Wienerberger realized they need to address and optimize the high energy consumption of the production processes in their plants. As a consequence, the key question they asked was: how can we leverage smart energy management to reduce operational costs and the carbon footprint of our production processes?
Challenges
For most manufacturing firms like Wienerberger, fully understanding the energy consumption of the production processes is already a challenge, but controlling, predicting, and optimizing it is an even bigger hurdle to tackle. Instead of relying solely on operator intuition, they should use a data-driven solution to improve their manufacturing processes. With regard to this, there are multiple challenges that Wienerberger faced when trying to understand the (controllable) drivers of their energy-intensive production processes.
First of all, producing roof tiles and bricks is a slow, continuous process that involves different types of materials. Moreover, the process uses a lot of energy which, due to inflated energy prices, is very costly. Second, in order to measure the process variables that could have an impact on the energy consumption of the production processes, Wienerberger employs over 100 different sensors that gather data on these variables. Besides sensor data, other data sources such as shop floor data, external data, inventory data… are available to use in building and training an AI model. Although collecting data is a necessary first step in gaining insight into the energy consumption, preprocessing these raw data is a crucial next step to really achieve this objective. In this case the plant was older and the data, not yet centralized, available but in 40 thousand separate CSV files. Therefore, an extensive cleaning of the data, parsing for multiple formats, and selecting the data with the best predictive power are some of the required preprocessing tasks.
Third, Wienerberger faced the challenge that not all properties of the oven state could be measured directly. In order to fully control and reduce the energy consumption of their production processes, they could not ignore these variables just because they can’t be measured directly. After all, these process variables could have an influence on the energy consumption and so they should be taken into account as well. As a consequence, digital twins simulations that act as virtual sensors should be created to measure these process variables.
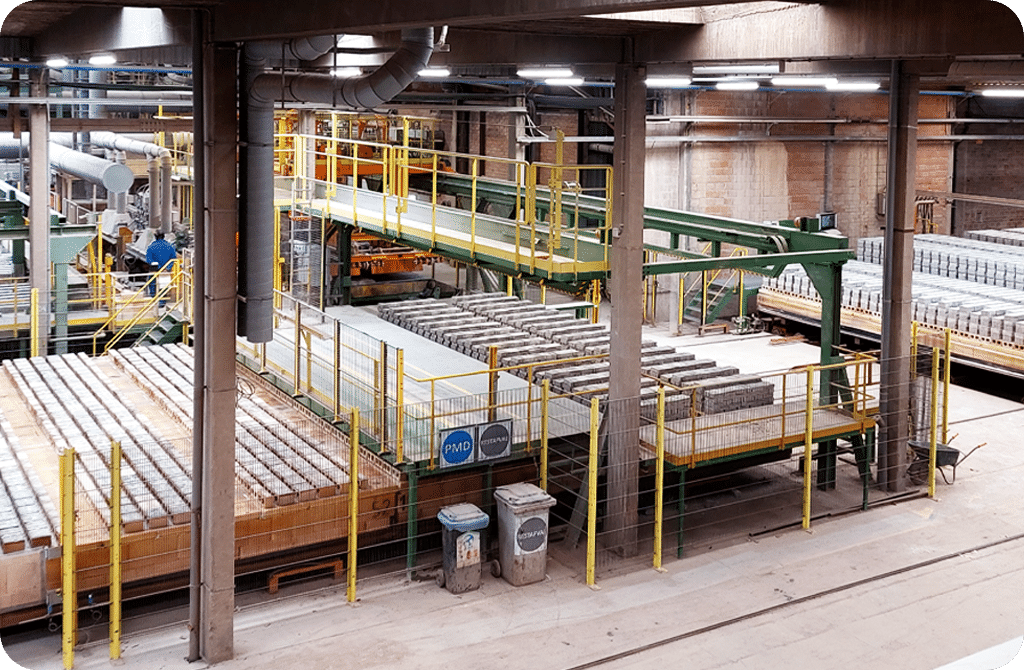
Solution
At Faktion, we heavily focus on Smart Manufacturing (SMF) and its three main fields (Process Optimization, Equipment Efficiency, and Smart Planning), and have a dedicated SMF Engineering & Delivery team that is knowledgeable on the process industry. Over the years, we have helped numerous clients in their Smart Manufacturing projects, hence we were able to quickly identify the key parameters and features that influence the outcome.
During the project at Wienerberger, we used an agile and proactive collaboration with Wienerberger's SMF Team in order to make the right assumptions. After all, it is important to deeply understand the process, and recognize the production process in the data, before we are able to improve it. Furthermore, there is an emphasis on knowledge transfer between the Faktion team and Wienerberger's process engineers to ensure optimal value creation. Faktion uses a data-centric approach, starting with a Proof of Concept (POC) on a subset of the data followed by a Proof of Value (POV) on the full static and historical dataset.
Before using Machine Learning (ML) techniques to analyze the data, the data had to be put in a proper format. Besides a thorough pre-processing of the 40k CSV files to create the initial dataset, soft sensors (digital twins) were used to model important datapoints that were not captured in the manufacturing process. By building this digital twin, you improve the data quality and availability before it is run through a ML pipeline, ultimately leading to better results.
After putting the data in the right format for analysis, our team provided a quantification of all influences on gas consumption in the baking and drying processes using time series analysis and different types of regression models.
This gave the process engineers at Wienerberger a reliable model that takes into account the material properties (amount, density and chemical composition), process settings (speed of kilns, target temperature, …), and environment characteristics (outdoor temperature and humidity). The model manages to capture the 10-15 main (controllable) drivers of energy consumption, explains 92 percent of the variance, and allows Wienerberger's engineers to understand, simulate, and optimize the energy consumption of the production processes.
This data-centric approach shows how important it is to put the data first. In the early phases of the project, business value can already be created (minimizing energy consumption) with insights from a static offline POV. In further phases of the project, the model is deployed in Wienerberger's operating environment and afterwards scaled across the whole organization.
Results and Going Further
Combining and analyzing the vast amount of data that was gathered in the manufacturing facility enabled Wienerberger to perform smart energy management, bringing them several benefits. Overall, the process engineers at Wienerberger have gained an increased understanding in the energy consumption drivers. As a result, they now have the capability to reduce the energy consumption in their plant without compromising on product quality. Furthermore, the solution in this plant can be upscaled to other Wienerberger facilities. Not only does this lead to a significant reduction of the carbon footprint of the production processes, it also helps Wienerberger to decrease the operational costs as the high energy and gas prices had turned the energy consumption into an even larger cost. Another related benefit is an improved forecasting of the production costs of the baking and drying processes at their manufacturing facilities. Looking into the future, the solution we developed in collaboration with Wienerberger lays the foundation for later AI initiatives. For example, this data could be used to explain anomalies due to e.g. batch-to-batch variation, or doing a golden batch analysis for energy consumption on a specific brick type.
